Prediction of PM2.5 using Micro-satellite Imagery and Sensor Ambient Air Quality Monitor Network (SAAQM)
Prediction of PM2.5 at 1 km × 1 km or maybe less at sub-km levels will enhance the spatial distribution over the study area. Recent literature and studies have shown that integrating AQ monitors data with satellite imagery using deep learning methods holds great promise in improving exposure estimates and pinpointing APH. This approach can also be used in plume detection and identification of point sources of air pollution from space.
Research Methodology
The government air quality monitors are present very sparsely in the cities. The installation and operation of new monitoring stations requires a lot of efforts and monetary resources. The study will include the deployment of the SAAQM network, which will increase the dense sensor network for increasing the spatial heterogeneity of PM2.5 along with a limited number of governments’ monitors available in the area. SAAQM network will be integrated with satellite imagery.
Commercial satellites from Planet Labs, a constellation of ~200 dove satellites revolving around the globe, provide daily satellite images (3-band) with a high resolution of ~3.7 m/pixel. These satellite images will be downloaded over our SAAQM + continuous ambient air quality monitoring stations (CAAQMS) locations in 1 km × 1 km tiles and labelled with ground truth PM2.5 values and meteorological parameters. This high-resolution data will be used to develop machine-learning models to predict PM2.5. The procedure will also use various artificial intelligence and deep learning techniques. Some of them include image augmentation, image segmentation, random forest, CNN and other joint models. Predicted PM2.5 at 1 km × 1 km or maybe less at sub-km levels (200m, 300m, 500m, 700m) spatial resolution will enhance the spatiotemporal distribution over the city/study area. Recent literature and studies have shown that integrating low-cost ground monitors with satellite imagery using deep learning methods holds great promise in improving exposure estimates and pinpointing APH. This approach can also be used in plume detection and identification of point sources of air pollution from space.
Future Applications
1. Spatial maps of PM2.5 at sub-km levels (200m, 300m, 500m, 700m etc.)
2. Reach to remote and rural areas, where no monitoring is possible
3. Smoke-plume detection using satellite imageries
4. Spatial information about the exposure levels and health risk estimates
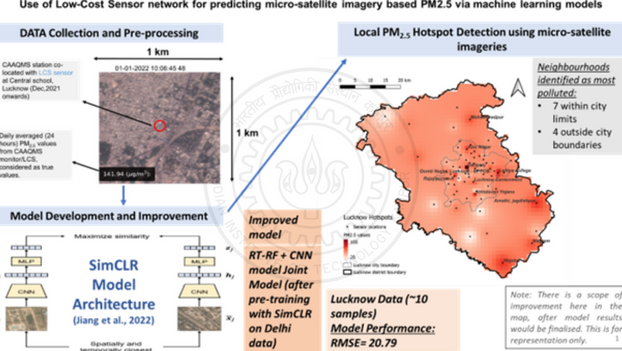